Your #1 Ethical AI List
Your one-stop-shop for the best AI model options!
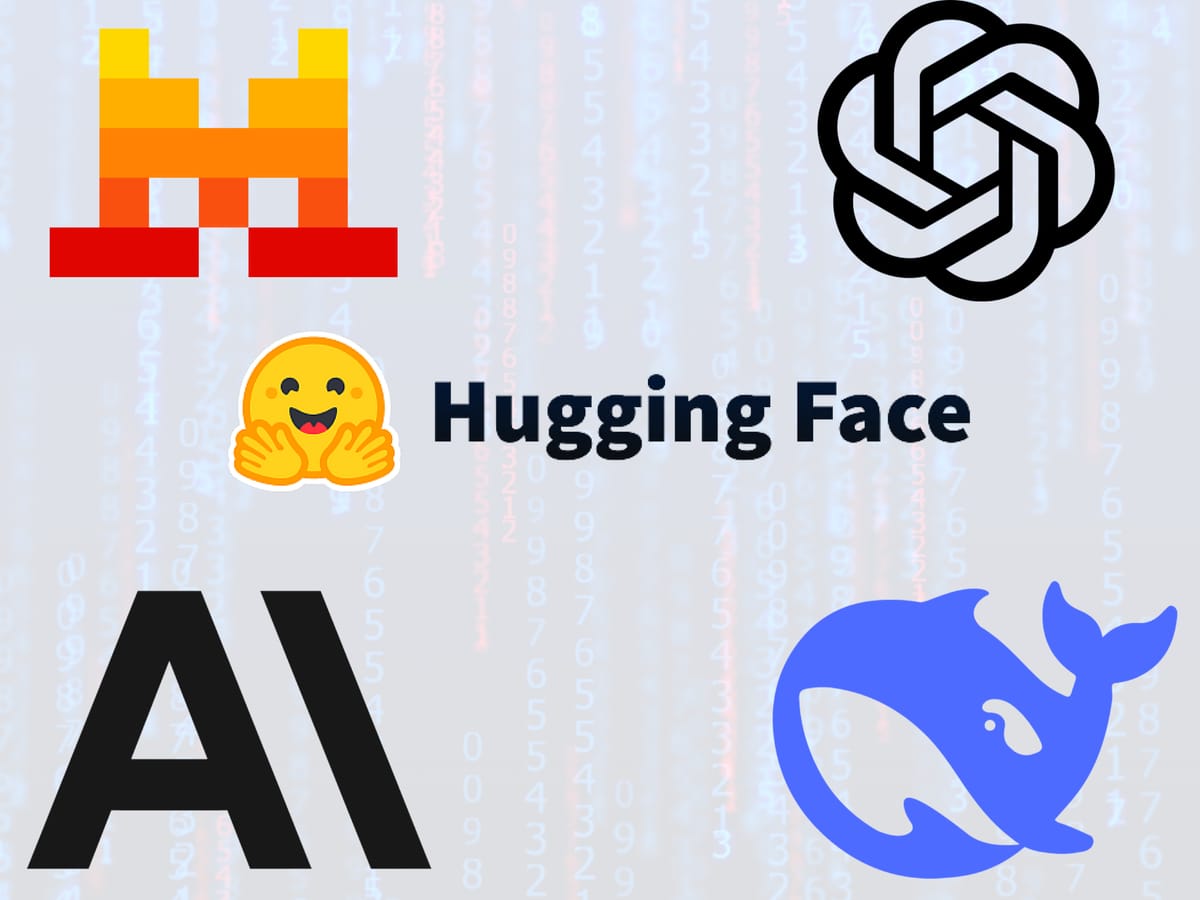
In the piranha feeding frenzy of tech bro one-upmanship that is the AI news cycle, you might be finding it hard to figure out which of these tools you should actually use. But what if the equation was made simple? What if your choice of AI was grounded in harm reduction?
I recently talked with someone about the harms of AI, particularly in the environmental real, and they were surprised to learn that AI had an incredibly high environmental impact. Likewise, further conversation revealed that they were only lightly aware of the true damage behind the illegal and unethical use of author and artist's work as raw training data for these models.
Until our conversation, this person had almost no idea that there were problems with AI. That's why I'm writing this guide.
- In part one, we'll learn a little about some of the challenges with AI.
- In part two, I'll present my list of the best companies and organizations to use for AI services.
Part One: Understanding the problem
Let's start with some stats. As of March 2024, OpenAi's ChatGPT tool uses over half a million kilowatts of electricity every day. That's 180,000 households, or 75.6% of the households in all of Wyoming [^1]. That's a single AI company. When the OpenAI super-data center is complete in 2028, we can expect that number to have climbed astronomically.
Furthermore, we know that 5 simple ChatGPT queries use as much water a 16-oz bottle 1. These are tasks like ranking favorite movies or reordering a short list. Generative tasks (like text and image generation) use more water per query.
When you line all these problems up in a row it becomes reasonable to ask: is an "ethical" AI possible at all?
And the issues don't stop at environmental impacts. A 2024 meta-study "identified and categorized significant risks of emerging ChatGPT-like tools at the individual, organizational, and societal levels." 2 These risks include:
- AI increases the proliferation of realistic disinformation.
- AI fosters an increase in realistic scams.
- AI allows harmful targeted personal attacks to be automated.
- AI is contributing to human social isolation.
- AI is displacing human workers across multiple sectors.
- AI increases perils to businesses in terms of security and privacy.
- AI fosters weakened critical intellectual abilities (and devalues education credentials).
- AI may offer generous free options now, but stratification of access will create significant digital (and therefore physical) inequities.
- AI in the medial sector shows serious risks of system errors and confidentiality.
Alongside these issues, there are also devastating consequences for artists and authors whose work has been used (without permission) to train these models. Meta (currently flailing through a massive lawsuit regarding illegally downloaded and stolen novels used for training) is a particularly egregious example.
When you line all these problems up in a row, it becomes reasonable to ask: is an "ethical" AI possible at all?
The answer is complex.
The United Nations and the European Union are currently pushing hard for ethical standards in AI development and use. China is pivoting from pure development efforts towards ethical scaffolding. Only the United States is failing to make significant and reasonable headway in this area.
But we can not ignore the power and usefulness of AI. Tasks that were once specialized are slowly opening up to those with entry-level skills. There's a beautiful potential for the democratization of skill access through AI tools. Moreover, for those within a corporate structure, understanding how to use AI will soon be a vital job skill. For all of these reasons, it's important to take a step back and explore those tools currently available which offer something approaching ethical norms.
Part Two: Ethical AI Tools
Sure! Here are the companies formatted without the footnote markers:
╔══════════════╗
║ MISTRAL AI ║
╠══════════════╣
║ 🌱 Env: Efficiency focus ║
║ 👥 Labor: No large-scale human outsourcing ║
║ 🧠 Data: Open datasets ║
║ 🏛️ Gov: Open weights ║
╚══════════════╝
Category | Details |
---|---|
Environmental Impact | Emphasizes efficiency in model design to reduce compute needs [^3]. No disclosed carbon or water usage; trained using the European High-Performance Computing infrastructure (which means a likely lower-carbon energy footprint) [^4]. |
Worker Rights & Labor | No large-scale outsourcing reported; initial model built without a costly human reinforcement stage, limiting reliance on underpaid and disadvantaged populations. |
Data Sourcing & Copyright | Uses open datasets for fine-tuning (no proprietary data) [^3], but has not fully disclosed pre-training sources [^4] (so there is a large likelihood of copyrighted content). |
Governance & Power | Open-sourced model weights (Apache 2.0 license) [^5] helps promote transparency. Traditional Venture Capital-funded startup governance though -- never a great option for ethical choices. No known ethical oversight board. |
╔══════════════╗
║ ANTHROPIC ║
╠══════════════╣
║ 🌱 Env: High energy use ║
║ 👥 Labor: RLHF via contractors ║
║ 🧠 Data: Scraped corpora ║
║ 🏛️ Gov: Public Benefit Corp ║
╚══════════════╝
Category | Details |
---|---|
Environmental Impact | Trained very large models (52B+ parameters) with estimated energy use of ~1.3 GWh and hundreds of thousands of liters of water [^6]. Does not publicly report its own footprint, but Google (its cloud provider) claims 100% renewable energy matching [^6]. Google's not exactly known for its ethical behavior, though. |
Worker Rights & Labor | Relied on contractors for RLHF (Reinforcement Learning from Human Feedback), but has been sketchy about the number of workers and how they get paid [^7]. Created a process called "Constitutional AI" to reduce reliance on human labelers and limit their exposure to toxic content [^8]. |
Data Sourcing & Copyright | Training data not disclosed [^6]; likely includes large-scale internet scraping, including from illegal sources. Now facing lawsuits from authors and publishers [^9]. No known consent from data sources. Claims fair use, which is reasonably absurd, but is being debated in the courts. |
Governance & Power | Structured as a Public Benefit Corporation. Overseen by a Long-Term Benefit Trust designed to prioritize public good [^10]. A majority of board members will ultimately be elected by the trust [^11], making it a really neat attempt to avoid a profit-motive-centered governing structure. |
╔══════════════╗
║ GROQ ║
╠══════════════╣
║ 🌱 Env: Energy-efficient LPUs (AI processors) ║
║ 👥 Labor: No outsourcing ║
║ 🧠 Data: Open models ║
║ 🏛️ Gov: VC-funded hardware ║
╚══════════════╝
Category | Details |
---|---|
Environmental Impact | Focuses on AI chip design: their LPUs (Language Processing Units) are up to 10× more energy-efficient than traditional GPUs [^12]. This is a huge win for the environment. While model training still typically uses GPUs, Groq significantly reduces the environmental cost of running those models [^13]. |
Worker Rights & Labor | Does not outsource data annotation to exploited laborers. As a hardware infrastructure provider, Groq is doesn't actually focus on building models. Their internal workforce primarily consists of engineers under standard tech industry practices. |
Data Sourcing & Copyright | Primarily runs open models like Meta’s LLaMA-2 and Mistral [^13]. Important to note that while Meta's models are largely open-source (good), everything else about that company is unethical as heck. Groq themselves do not build proprietary datasets or scrape data. Operates within license terms of hosted models and avoids copyright disputes by using only pre-existing models [^13]. |
Governance & Power | Traditional Venture Capital-funded hardware startup (again, VC isn't great usually). Promotes accessibility through both cloud and on-premises deployment [^13] meaning it's possible to buy the Groq LPU chips and run powerful AI locally. They work with the open-source AI community but have no formal ethical oversight structures. Governance is executive- and investor-driven (not great). |
╔══════════════╗
║ HUGGING FACE ║
╠══════════════╣
║ 🌱 Env: Climate impact focus ║
║ 👥 Labor: Volunteer-driven ║
║ 🧠 Data: Responsible sourcing ║
║ 🏛️ Gov: Ethics & Society team ║
╚══════════════╝
Category | Details |
---|---|
Environmental Impact | Co-led the BLOOM project (176B parameters) and publicly released its carbon footprint—approximately 50 metric tons of CO₂ for future AI training [^14]. Invests in model efficiency research and advocates for model sharing to reduce redundant compute. |
Worker Rights & Labor | Avoids exploitative labor practices. Major collaborative projects like BigScience were volunteer-driven [^15], with contributions from global researchers rather than low-paid crowd labor. Promotes an inclusive, community-oriented workplace culture. |
Data Sourcing & Copyright | Strong emphasis on data ethics. The ROOTS corpus for BLOOM was carefully sourced with respect for original licenses [^15], documentation, multilingual representation, and consent. Hugging Face removed problematic datasets when privacy concerns were raised. That said, they do host plenty of unethically-trained models, like LLaMA. |
Governance & Power | Champions “responsible openness.” Hosts a visible Ethics & Society team [^16], shares updates on governance, and engages with public policy. Executives retain power as a private company, but business model aligns with community trust and openness. |
╔══════════════╗
║ DEEPSEEK ║
╠══════════════╣
║ 🌱 Env: Intensive compute ║
║ 👥 Labor: Model distillation ║
║ 🧠 Data: Opaque sourcing ║
║ 🏛️ Gov: Low transparency ║
╚══════════════╝
Category | Details |
---|---|
Environmental Impact | Training details for DeepSeek-R1 are not public, but the model is presumed to require high compute to reach its performance level [^17]. Operates in China, where the energy grid is still heavily carbon-intensive despite recent efforts to go green. No disclosed mitigation strategies, despite growing concern in Chinese government over AI’s energy footprint [^18]. |
Worker Rights & Labor | Apparently developed R1 using "model distillation," which means it was trained on outputs from other AIs instead of relying on large-scale human labeling [^18]. This reportedly cut costs to millions instead of billions [^19], suggesting less exposure to labor exploitation. However, lack of transparency and local context make it difficult to verify. |
Data Sourcing & Copyright | Released as a fully open-weight model [^19], but training data sources remain undisclosed. Accused of using output from ChatGPT without permission [^18] (oh irony of ironies! OpenAI accuses other companies of stealing copyrighted data when it ha stolen the whole corpus of human knowledge). There has been an analysis suggesting OpenAI content was included [^20], though since large portions of the Internet have now been OpenAI generated, who's to say. |
Governance & Power | Operates as a Chinese startup with minimal transparency. Provided insufficient data-use details to EU regulators, resulting in an Italy ban [^20]. Allegedly follows Chinese state content rules and resists European legal oversight [^20]. Governance appears top-down, with accountability only to internal leadership. |
Which is the best? TLDR; HuggingFace
So, from this list, we have a clear winner: HuggingFace! They offer a massive range of models for free (you can even download many of them and run them on your own computer). They've got the most ethical structure, and they've also attempted to support research into greening AI.
Now, that said: Mistral and Groq are both pretty reasonable options as well. Both offer reasonable free versions.
Groq is especially nice for anyone who wants API access, as that is incredibly cheap. But it's not as well-rounded an offering (no internet access).
Mistral's Le Chat is cheaper than ChatGPT, has a generous free tier. It's also pretty powerful and features image generation, Internet search, a Canvas-feature, the best OCR in the business, and more to come!
However, if you really need power and don't want to buy into OpenAI, your best bets are Anthropic or DeepSeek. That said: DeepSeek has definitely raised flags with its data privacy issues and general lack of transparency. If you want to use their models, I'd recommend just downloading one of the smaller quantized versions and playing with it locally (you'll need 64GB of ram and a 24GB GPU minimum).
Which leaves Anthropic as the best OpenAI contender. They're still not great, but they've at least gone a bit farther than other tech-bro VC-funded giants in trying to build ethics into their core operations.
Bonus: If you really need a powerful Internet search tool your best bet is Perplexity. I haven't looked into their ethical and legal situation yet, but their basic search is free and useful (just be careful as it tends to also have a lot of sneaky biases). Or, if you need to do some heavy research, check out Elicit -- their free tool's solid and is a great way to start a search into an unfamiliar topic.
So, there you have it! I'll be continuing to write and think about AI topics in the future. Want me to look into something for you and use my awesome librarian skills for the betterment of humanity? Leave a comment and I'll see what I can do!
👋 Hi there! I’m Odin Halvorson, a librarian 📚, independent scholar 📖, film fanatic 🎬, fiction author 📝, and tech enthusiast 💻. If you like my work and want to support me, please consider becoming a paid subscriber to my newsletter for as little as $2.50 a month! 📣
Support me in other ways:
Start writing and reading with the Supernote, and support me at the same time! Like audiobooks? Hate DRM? Use this affiliate link to sign up for Libro.fm, where you actually own the books you buy! 🎧 Want your own Ghost newsletter? Check out MagicPages for the cheapest hosting rates via my affiliate link (they even offer lifetime hosting plans!) 📈
Thanks for your support! 🙏
References
[^1]: Kshetri, Nir. “The Environmental Impact of Artificial Intelligence.” IT Professional 26, no. 3 (May 2024): 9–13. https://doi.org/10.1109/MITP.2024.3399471.
[^2]: Polyportis, Athanasios, and Nikolaos Pahos. “Navigating the Perils of Artificial Intelligence: A Focused Review on ChatGPT and Responsible Research and Innovation.” Humanities and Social Sciences Communications 11, no. 1 (January 15, 2024): 1–10. https://doi.org/10.1057/s41599-023-02464-6.
[^3]: Albert Q. Jiang et al. “Mistral 7B.” arXiv preprint, October 10, 2023. https://arxiv.org/abs/2310.06825.
[^4]: Center for Research on Foundation Models. “Mistral 7B—Transparency Report.” Foundation Model Transparency Index, May 2024. https://crfm.stanford.edu/fmti/May-2024/company-reports/Mistral_Mistral 7B.html.
[^5]: Mistral AI. “Announcing Mistral 7B.” Blog post, October 10, 2023. https://mistral.ai/en/news/announcing-mistral-7b.
[^6]: Credo AI. “Anthropic Claude—AI Vendor Risk Profile.” AI Vendor Directory, July 31, 2023. https://www.credo.ai/ai-vendor-directory/anthropic-claude.
[^7]: Dzieza, Josh. “AI Is a Lot of Work.” New York Magazine, June 20, 2023. https://nymag.com/intelligencer/article/ai-artificial-intelligence-humans-technology-business-factory.html.
[^8]: Anthropic. “Constitutional AI: Harmlessness from AI Feedback.” Research paper, December 15, 2022. https://www.anthropic.com/news/constitutional-ai-harmlessness-from-ai-feedback.
[^9]: Brittain, Blake. “Anthropic Fires Back at Music Publishers’ AI Copyright Lawsuit.” Reuters, January 17, 2024. https://www.reuters.com/legal/litigation/anthropic-fires-back-music-publishers-ai-copyright-lawsuit-2024-01-17/.
[^10]: Perrigo, Billy. “Inside Anthropic, the AI Company Betting That Safety Can Be a Business Model.” Time, July 25, 2024. https://time.com/6980000/anthropic/.
[^11]: Perrigo, Billy. “How Anthropic Designed Itself to Avoid OpenAI’s Mistakes.” Time, July 28, 2024. https://time.com/6983420/anthropic-structure-openai-incentives/.
[^12]: AI IXX. “Groq AI Chips vs. Nvidia: A Comparative Analysis.” AI IXX blog, October 11, 2024. https://aiixx.ai/blog/groq-ai-chips-vs-nvidia.
[^13]: Groq. “Groq Is Fast AI Inference.” Accessed April 17, 2025. https://groq.com/.
[^14]: Luccioni, Alexandra Sasha, Sylvain Viguier, and Anne‑Laure Ligozat. “Estimating the Carbon Footprint of BLOOM, a 176B‑Parameter Language Model.” Journal of Machine Learning Research 24, no. 253 (2023): 1–15. https://jmlr.org/papers/volume24/23-0069/23-0069.pdf.
[^15]: Laurençon, Hugo, et al. “The BigScience ROOTS Corpus: A 1.6 TB Composite Multilingual Dataset.” OpenReview, March 7, 2023. https://openreview.net/forum?id=UoEw6KigkUn.
[^16]: Hugging Face. “Society & Ethics at Hugging Face.” Accessed April 17, 2025. https://huggingface.co/society-ethics.
[^17]: Evans, Kieran. “DeepSh*t: Exposing the Security Risks of DeepSeek‑R1.” HiddenLayer blog, February 12, 2025. https://hiddenlayer.com/innovation-hub/deepsht-exposing-the-security-risks-of-deepseek-r1/.
[^18]: University of Michigan News. “Up to 30 % of the Power Used to Train AI Is Wasted—Here’s How to Fix It.” November 10, 2024. https://news.umich.edu/up-to-30-of-the-power-used-to-train-ai-is-wasted-heres-how-to-fix-it/.
[^19]: DiEM25 Communications. “A Better Approach for Building Ethical AI in Light of DeepSeek News.” DiEM25, March 12, 2025. https://diem25.org/a-better-approach-for-building-ethical-ai-in-light-of-deepseek-news/.
[^20]: Lakshmanan, Ravie. “Italy Bans Chinese DeepSeek AI Over Data Privacy and Ethical Concerns.” The Hacker News, January 31, 2025. https://thehackernews.com/2025/01/italy-bans-chinese-deepseek-ai-over.html.
Bibliography
Mistral AI. Le Chat (large language model interface). Accessed April 17, 2025. https://chat.mistral.ai. Used to support formatting, particularly with block sections and footnotes.
OpenAI. ChatGPT-4o (multimodal AI model via ChatGPT). Accessed April 17, 2025. https://chat.openai.com. Used to supplement own research tools and present information which was then human-reviewed and rewritten.